We have a soft spot for long, thorough guides here at TDS—but we also appreciate focused posts targeting specific challenges and pain points that data scientists face in their day-to-day work.
To celebrate these highly useful and practical articles, this week’s Variable turns the spotlight on recent highlights from our Tips & Tricks column: they offer actionable, tried-and-true advice that can help you save time and effort and produce better results in your projects. Whether or not you’ve already enjoyed your share of treats this week (happy belated Halloween to those who celebrate!), we hope these tricks will inspire you to find a new approach or tool to experiment with.
- Streamlining Repetitive Tasks During Exploratory Data Analysis
EDA sometimes gets a bad rap for being the tedious stage you have to plough through to reach the more interesting stages of modeling and prediction work. Christabelle Pabalan recently shared a smart approach that adds a layer of automation to the process—but without sacrificing care and precision along the way. - Explore Pydantic V2’s Enhanced Data Validation Capabilities
Pydantic, “the most widely used data validation library for Python,” is a go-to tool for many data practitioners. Lynn Kwong’s overview of Pydantic V2 provides concrete tips on making the most of its latest improvements, which include supporting strict mode and the possibility to validate data without a model.
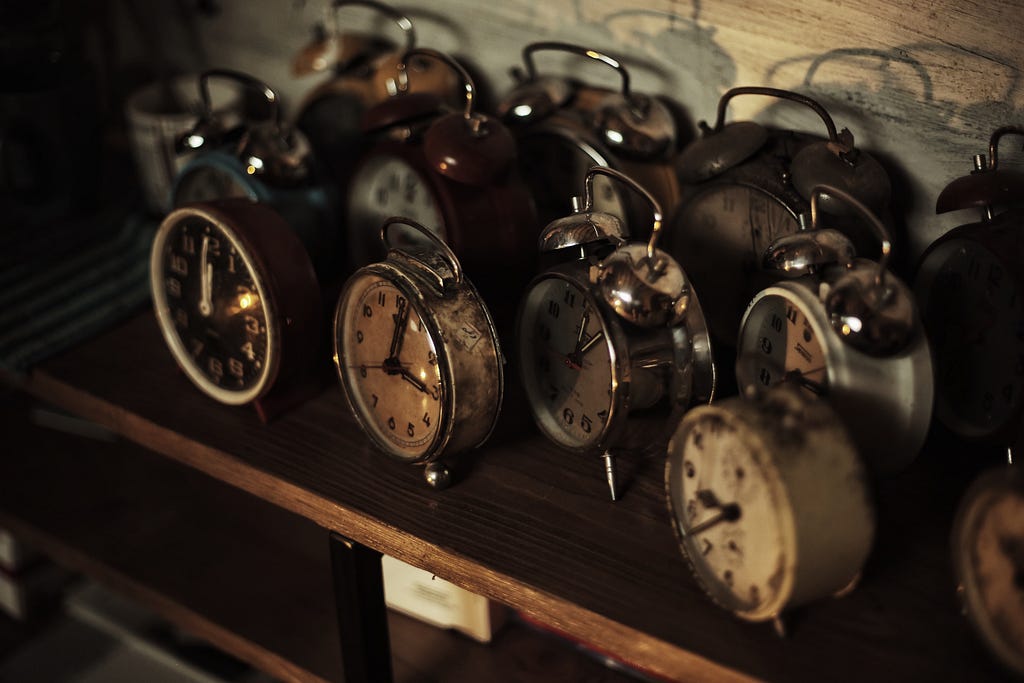
- 6 Common Index-Related Operations You Should Know about Pandas
Given Pandas’ ubiquity in data-science workflows, it’s never a bad idea to gain a deeper understanding of its features and expand your knowledge of effective ways to handle dataframes. Yong Cui’s new post zooms in on index-related operations and breaks them down using simple, real-life use cases as examples. - How to Use Color in Data Visualizations
If you’ve been treating color choices in your charts and plots as an afterthought, Michal Szudejko’s compendium of tips on the proper use of color is sure to make you reconsider your approach. From accessibility to palette options, you’ll learn how small tweaks can make your visualizations clearer and help them become stronger storytelling tools. - Unleashing the Power of the Julia SuperType
For the growing number of Julia aficionados out there, Emma Boudreau’s hands-on resource on abstraction and how to incorporate it effectively into your code is a must-read—it offers a detailed overview of the ways you can start creating our own supertypes with minimal effort.
We hope you’ve still got room for a few extra treats, because we wouldn’t want you to miss these excellent reads on other topics:
- How will the proliferation of AI-generated content affect the quality of LLM training down the line? Aicha Bokbot explores an emerging concern around the sustainable development of AI tools.
- Music meets machine learning in Emmanouil Karystinaios’s fascinating project, which attempts to automate harmonic analysis.
- Interested in building and publishing an R data package? Deepsha Menghani offers a step-by-step guide that leverages devtools for accomplishing this goal.
- Using hybrid search, hierarchical ranking, and instructor embedding, Agustinus Nalwan attempts to address a major challenge in the use of RAG in domain-specific searches.
- For a lucid reflection on the current state of the AI startup ecosystem, don’t miss Clemens Mewald’s recent deep dive, which explains why LLMs have succeeded in going mainstream whereas MLOps tools have not.
- Questionable data-hacking practices are, sadly, all around us; Hennie de Harder offers a helpful look at the statistics concepts behind them.
Thank you for supporting the work of our authors! If you enjoy the articles you read on TDS, consider becoming a Medium member — it unlocks our entire archive (and every other post on Medium, too).
Until the next Variable,
TDS Editors
Had Your Treats? Time for Data Science Tricks was originally published in Towards Data Science on Medium, where people are continuing the conversation by highlighting and responding to this story.